Zuletzt aktualisiert am 4. Mai 2025
Between 2022 and 2023, approximately 15 billion AI-generated images were created. However, the quality of these visuals depends on the image databases they draw from. A new study reveals that AI tends to reinforce gender stereotypes in representations of technical professions.
Text-to-image generators like DALL·E Mini can create realistic images from simple text prompts. Yet aspects of diversity are often overlooked. AI image generators such as DALL·E Mini reinforce gender and ethnic stereotypes by systematically underrepresenting or misrepresenting women and people of color. Tim Breuer’s bachelor’s thesis illustrates that this bias extends to portrayals of technical professions: out of 180 images generated with DALL·E Mini, only 35 featured women and just 7 included people of color. Even prompts designed with diversity in mind are only partially effective in reducing this bias.
STEM Professions Through the Lens of Generative AI
The study centers on job-related images generated specifically for roles in the STEM fields—science, technology, engineering, and mathematics. In computer science and engineering in particular, the AI model predominantly produced figures constructed as white and male. This outcome is driven not only by the limited scope of training data, as highlighted by DALL·E Mini developer Boris Dayma in his technical report, but also by broader systemic issues such as the lack of ethical guidelines in AI, absent legal regulations, and the low level of diversity within development teams.
DALL·E Mini Fails to Represent Women in Tech and IT Roles
The job descriptions used in the study were written in English, as the model is optimized for that language, according to its developer Boris Dayma. Additionally, the grammatical gender inherent in the German language could influence output. Due to the origin of the training data, U.S. occupational demographics were used for comparison. The findings revealed that DALL·E Mini completely excluded women from generated images for all computer science and engineering roles.
For positions like „Software Engineer,“ „Computer Scientist,“ or „IT Project Manager,“ only male-coded figures appeared—despite women making up between 20.2% and 33.6% of the actual workforce in these fields, according to the U.S. Bureau of Labor Statistics (2023) and Zippia Demographic Research (2024). A similar trend was observed in mathematics-related professions, with the exceptions of „Math Teacher“ and „Supply Chain Manager.“ Even in roles where women represent between 36.8% and 40.8% of workers, such as „Statistician“ or „Business Mathematician,“ the AI-generated images showed no female-coded figures. In total, only 35 out of 180 AI-generated images depicted women in STEM professions.
People of Color Almost Invisible in STEM Professions
The invisibility of people of color in the generated images was also striking. In all mathematics-related professions, such as „Accountant“ or „Math Teacher,“ where employment statistics show that between 26.6% and 40.6% of workers are people of color, DALL·E Mini only generated white-constructed figures. The same was true for computer science roles, where, except for „Software Engineer“ (11.1%), only white-constructed individuals were depicted. The bias was even more pronounced in the engineering sector. Despite people of color comprising up to 34.4% of workers in fields like „Engineer,“ „Electrician,“ or „Industrial Technician,“ the model consistently generated white-constructed figures. This resulted in an intersectional invisibility of both women and people of color in the tech sector. Overall, only 7 out of 180 analyzed AI-generated images featured people of color, indicating a significant bias in the image generation process.
AI-Generated vs. Actual Workforce Representation
In natural science professions like „Biologist“ or „Pharmacist,“ women were depicted more frequently, with representations ranging from 44.4% to 55.5%, bringing the AI-generated images closer to real-world distribution—a rare exception. This could be because these fields have a higher female representation in the training data, as natural sciences are seen as more attractive to women due to a focus on environmental and climate protection as a motivational factor. However, the general trend remains: women and people of color are further marginalized in STEM professions by AI-generated images. This disparity can be observed in a comparative table (Table 1: Female Representation; Table 2: PoC Representation*) between workforce demographic statistics and representation in DALL·E Mini.
Slider: Table 1 shows the percentage of female representation in the workforce for the selected twenty STEM professions (based on U.S. Labor Statistics and Zippia Demographic Research) compared to the representation in DALL·E Mini. Table 2 provides the same comparison for the PoC representation. /Source: Tim Breuer
Desensitized Development Processes
The causes of these biases primarily lie in the data used to train DALL·E Mini. The model relies on large image-text corpora like Conceptual Captions and Conceptual 12M, which are sourced from existing online image databases and websites. These sources are not neutral but reflect societal inequalities. DALL·E Mini, being a smaller model (specifically a „Mini“ version of DALL·E), became highly popular due to its simple usage terms as an open-access application, making it one of the first touchpoints for users with this technology.
A comparable study by Marc Cheong, a lecturer in Computer and Information Systems at the University of Melbourne, classifies figures generated by DALL·E Mini based on their „perceived gender“ (male, female, undefined) and their „perceived racial identity“ (white or non-white). This study also found that the AI predominantly generated figures constructed as either male or female, whereas the actual distribution is much more heterogenous. Furthermore, professions with a high percentage of female workers —such as „Waiter,“ „Baker,“ „Accountant,“ and „Judge“—were depicted with a lower proportion of female-constructed figures in the AI-generated images. This pattern is not exclusive to DALL·E Mini, as further research by Ranjita Naik and Besmira Nushi from the Microsoft Research team has shown that similar trends occur in other text-to-image generators.
Previous studies have shown similar patterns in other algorithmic systems. For instance, a 2015 study by researchers Amit Datta, Michael Carl Tschantz, and Anupam Datta revealed that Google advertisements for leadership positions in STEM professions were shown to men five times more likely than to women. Another study by scientist Tolga Bolukbasi (including Google DeepMind) in 2016 demonstrated that language models automatically associated occupations like „Computer Programmer“ with men and „Homemaker“ with women.
Initial Corrective Measures for a „Fairer AI“ Are Not Yet Convincing
The study also tested the technique of „Prompt Engineering“ to explore the feasibility and quality of more inclusive representation in AI-generated images. It was found that entering „black female engineer“ resulted in DALL·E Mini generating exclusively Black female engineers. While this method can partially mitigate biases, it also introduces new challenges. The generated images resembled stock photos, where the depicted individuals were often shown posing rather than in actual work scenarios. Additionally, it became evident that the model associates certain professions with specific image concepts. For example, when entering „computer scientist who is a woman,“ the model depicted women in lab coats working on computers—yet not as computer scientists. This suggests a misinterpretation based on learned word-image associations.
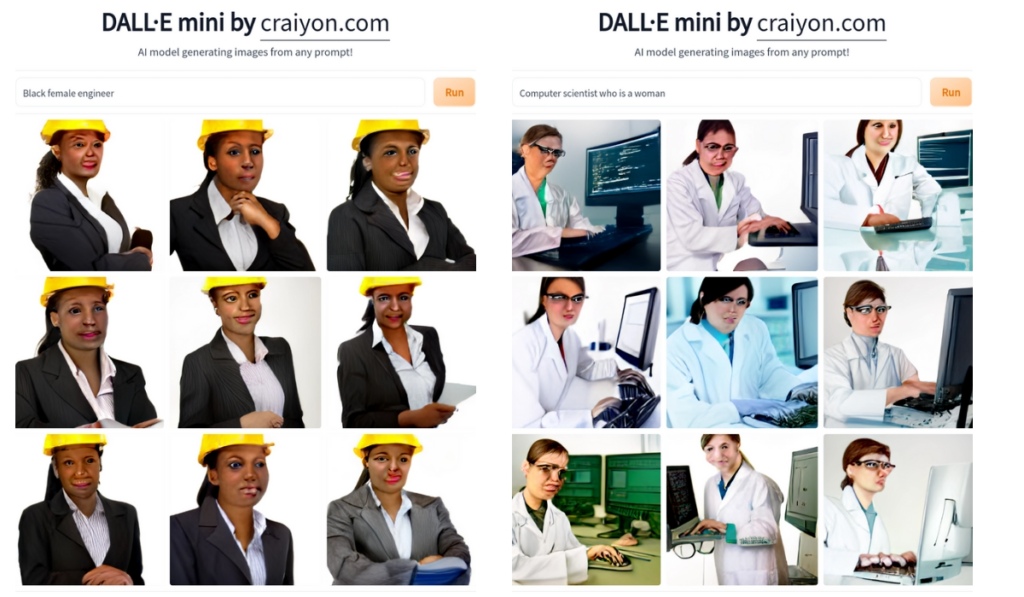
Another issue arises with current AI corrective measures. Google, in an attempt to increase diversity sensibility in AI image generators, made algorithmic adjustments. However, this led to flawed outcomes. Their „Gemini“ model, for example, depicted historical figures such as the American Founding Fathers and colonialists in a revisionist manner as Black-constructed. The U.S. media outlet Wired already observed that such types of corrections spark politically charged backlash around Diversity & Inclusion („DEI“). As a result, the structural problems in AI development are not yet sustainably addressed.
Ethics Teams for AI Development Processes
Interdisciplinary ethics teams could be more deeply involved in the AI development process to identify and minimize potential discrimination early on. Closer collaboration with the humanities could be beneficial in this regard.
Additionally, better labeling of AI-generated images could help raise awareness about potential biases. As AI systems are increasingly used to create media content, it is crucial that users are aware of the possible influencing factors. Training on AI ethics in media education could provide a solution to this.
About this study:
The study uses method of qualitative analysis based on Mayring to examine the representation of diversity in AI-generated images of STEM professions. For this, twenty English prompts were created, each generating nine images. The categories analyzed included gender attribution and people of color (PoC), focusing on visual features such as clothing, hairstyle, skin color, and physical traits. The image evaluation was conducted through manual coding based on a deductive coding guide. The results were organized in tables and qualitatively interpreted to assess potential biases and the influence of targeted linguistic adjustments (prompt engineering) on the portrayal of STEM professions.
A version of the paper was published as a scientific article in collaboration with Prof. Dr. Susanne Keil in the 55th issue of the Journal of the Women’s and Gender Studies Network NRW, focusing on the theme „Artificial Intelligence and Gender.“
/ This article was previously published in german and translated for our blog by Tim Breuer